While we try to keep things accurate, this content is part of an ongoing experiment and may not always be reliable.
Please double-check important details — we’re not responsible for how the information is used.
Civil Engineering
New Hybrid AI Tool Generates High-Quality Images Faster Than State-of-the-Art Approaches
Researchers developed a hybrid AI approach that can generate realistic images with the same or better quality than state-of-the-art diffusion models, but that runs about nine times faster and uses fewer computational resources. The tool uses an autoregressive model to quickly capture the big picture and then a small diffusion model to refine the details of the image.
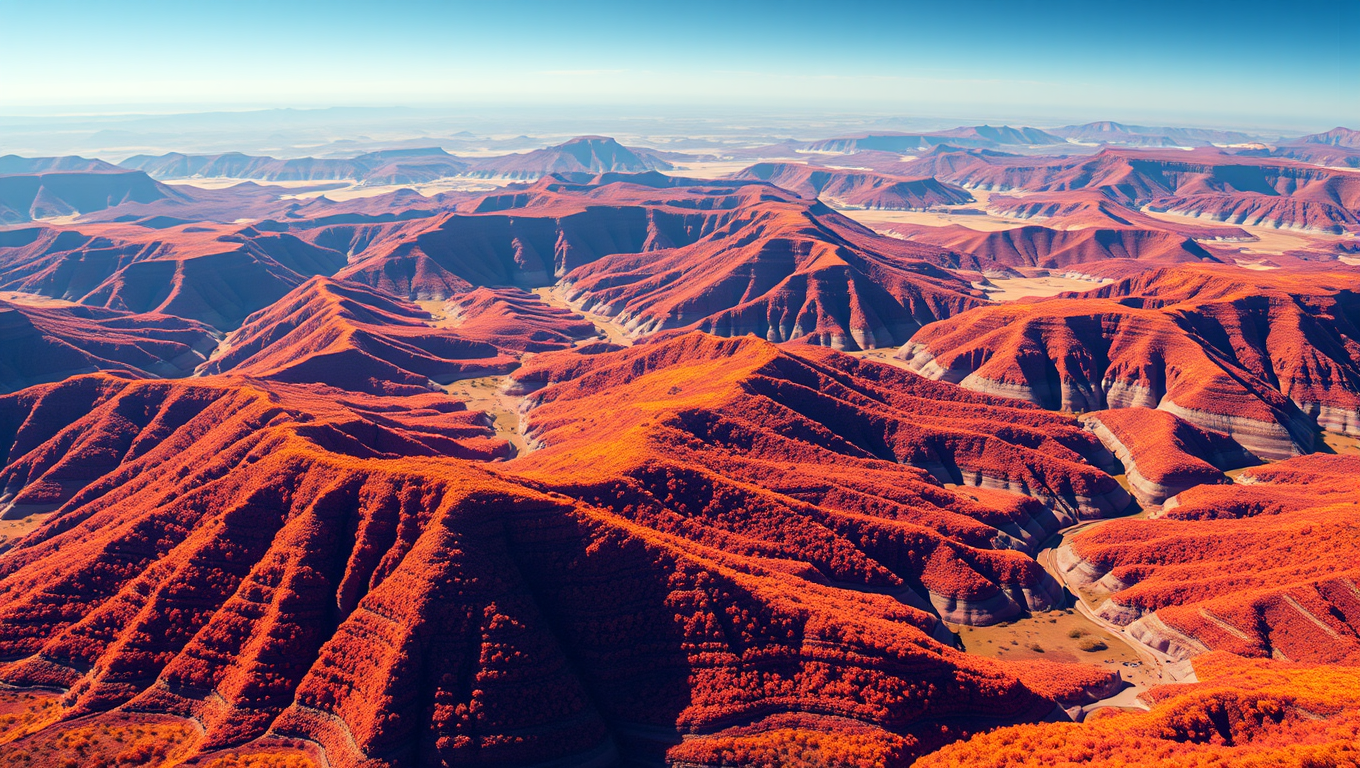
Biochemistry
A Game-Changing mRNA Vaccine that’s More Effective and Less Costly to Develop
A new type of mRNA vaccine is more scalable and adaptable to continuously evolving viruses such as SARS-CoV-2 and H5N1, according to a new study.
Batteries
Unlocking the Potential of Solid-State Batteries
Researchers have discovered that the mixing of small particles between two solid electrolytes can generate an effect called a ‘space charge layer,’ an accumulation of electric charge at the interface between the two materials. The finding could aid the development of batteries with solid electrolytes, called solid-state batteries, for applications including mobile devices and electric vehicles.
Ancient Civilizations
Reviving an Ancient Hue: Researchers Recreate Egyptian Blue Pigment
Researchers have recreated the world’s oldest synthetic pigment, called Egyptian blue, which was used in ancient Egypt about 5,000 years ago.
-
Detectors3 months ago
A New Horizon for Vision: How Gold Nanoparticles May Restore People’s Sight
-
Earth & Climate4 months ago
Retiring Abroad Can Be Lonely Business
-
Cancer4 months ago
Revolutionizing Quantum Communication: Direct Connections Between Multiple Processors
-
Agriculture and Food4 months ago
“A Sustainable Solution: Researchers Create Hybrid Cheese with 25% Pea Protein”
-
Diseases and Conditions4 months ago
Reducing Falls Among Elderly Women with Polypharmacy through Exercise Intervention
-
Chemistry4 months ago
“Unveiling Hidden Patterns: A New Twist on Interference Phenomena”
-
Albert Einstein4 months ago
Harnessing Water Waves: A Breakthrough in Controlling Floating Objects
-
Earth & Climate4 months ago
Household Electricity Three Times More Expensive Than Upcoming ‘Eco-Friendly’ Aviation E-Fuels, Study Reveals