Computational Biology
“Revolutionizing Sleep Analysis: New AI Model Analyzes Full Night of Sleep with High Accuracy”
Researchers have developed a powerful AI tool, built on the same transformer architecture used by large language models like ChatGPT, to process an entire night’s sleep. To date, it is one of the largest studies, analyzing 1,011,192 hours of sleep. The model, called patch foundational transformer for sleep (PFTSleep), analyzes brain waves, muscle activity, heart rate, and breathing patterns to classify sleep stages more effectively than traditional methods, streamlining sleep analysis, reducing variability, and supporting future clinical tools to detect sleep disorders and other health risks.
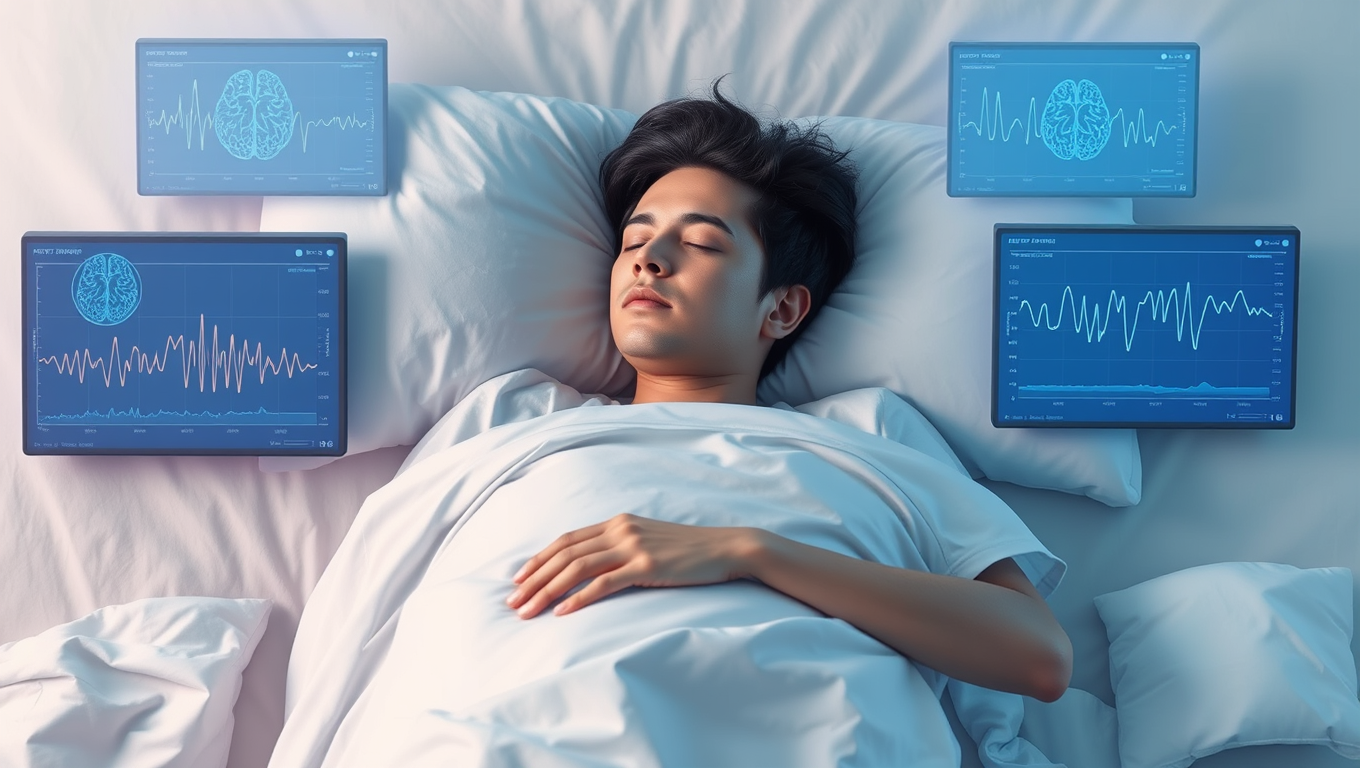
Chronic Illness
Unlocking the Power of Exposomics: Revolutionizing Health Science through Cutting-Edge Technology
Researchers in the field of exposomics explain how cutting-edge technologies are unlocking this biological archive, ushering in a new era of disease prevention and personalized medicine.
Computational Biology
“Dig Once” Approach to Upgrading Electrical and Broadband Infrastructure: A Cost-Effective Solution for Massachusetts Towns
When it comes to upgrading electrical and broadband infrastructure, new research shows that a ‘dig once’ approach is nearly 40% more cost effective than replacing them separately. The study also found that the greatest benefit comes from proactively undergrounding lines that are currently above ground, even if lines haven’t reached the end of their usefulness.
Computational Biology
Unlocking the Code: AI-Powered Diagnosis for Drug-Resistant Infections
Scientists have developed an artificial intelligence-based method to more accurately detect antibiotic resistance in deadly bacteria such as tuberculosis and staph. The breakthrough could lead to faster and more effective treatments and help mitigate the rise of drug-resistant infections, a growing global health crisis.
-
Detectors3 weeks ago
A New Horizon for Vision: How Gold Nanoparticles May Restore People’s Sight
-
Earth & Climate2 months ago
Retiring Abroad Can Be Lonely Business
-
Cancer2 months ago
Revolutionizing Quantum Communication: Direct Connections Between Multiple Processors
-
Agriculture and Food2 months ago
“A Sustainable Solution: Researchers Create Hybrid Cheese with 25% Pea Protein”
-
Diseases and Conditions2 months ago
Reducing Falls Among Elderly Women with Polypharmacy through Exercise Intervention
-
Albert Einstein2 months ago
Harnessing Water Waves: A Breakthrough in Controlling Floating Objects
-
Alternative Fuels2 months ago
Unlocking the Secrets of Supercritical Water: A New Understanding of Its Structure
-
Earth & Climate2 months ago
Household Electricity Three Times More Expensive Than Upcoming ‘Eco-Friendly’ Aviation E-Fuels, Study Reveals