While we try to keep things accurate, this content is part of an ongoing experiment and may not always be reliable.
Please double-check important details — we’re not responsible for how the information is used.
Communications
Enhancing Trust in Knowledge Work: How AI Can Boost Performance and Confidence
In a new study, researchers explored how artificial intelligence (AI) can enhance performance and trust in knowledge work environments. They found that when AI systems provided feedback in real-time, performance and trust increased.
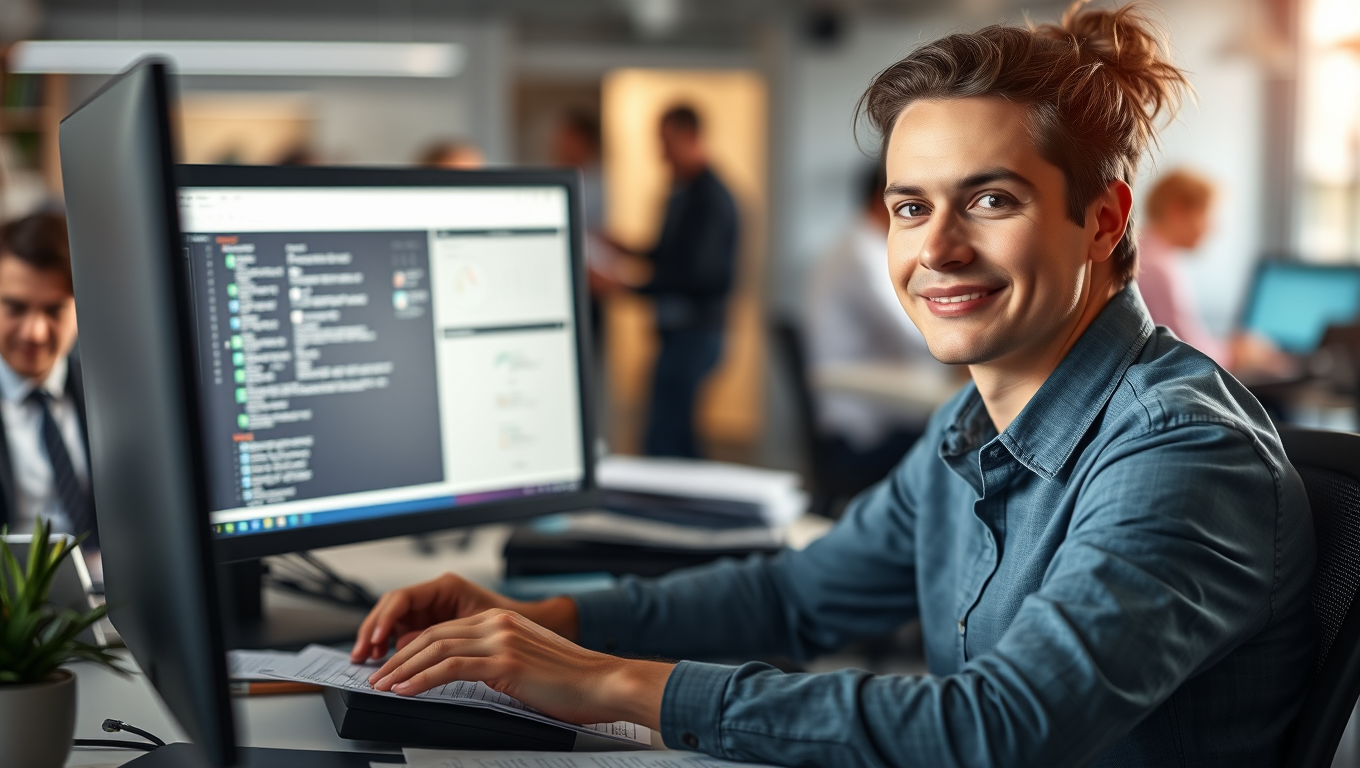
Communications
Artificial Intelligence Isn’t Hurting Workers—It Might Be Helping
Despite widespread fears, early research suggests AI might actually be improving some aspects of work life. A major new study examining 20 years of worker data in Germany found no signs that AI exposure is hurting job satisfaction or mental health. In fact, there s evidence that it may be subtly improving physical health especially for workers without college degrees by reducing physically demanding tasks. However, researchers caution that it s still early days.
Communications
Breaking Down Language Barriers in Quantum Tech: A Universal Translator for a Quantum Network
Scientists at UBC have devised a chip-based device that acts as a “universal translator” for quantum computers, converting delicate microwave signals to optical ones and back with minimal loss and noise. This innovation preserves crucial quantum entanglement and works both ways, making it a potential backbone for a future quantum internet. By exploiting engineered flaws in silicon and using superconducting components, the device achieves near-perfect signal translation with extremely low power use and it all fits on a chip. If realized, this could transform secure communication, navigation, and even drug discovery.
Communications
Scientists Take a Big Leap Toward the Quantum Internet with New Light Sources
A team of Danish and German scientists has launched a major project to create new technology that could form the foundation of the future quantum internet. They re using a rare element called erbium along with silicon chips like the ones in our phones to produce special particles of light for ultra-secure communication and powerful computing. With cutting-edge tools like lasers and nanotech, the researchers are working to make something that didn t seem possible just a few years ago: light that can both travel long distances and remember information.
-
Detectors3 months ago
A New Horizon for Vision: How Gold Nanoparticles May Restore People’s Sight
-
Earth & Climate4 months ago
Retiring Abroad Can Be Lonely Business
-
Cancer3 months ago
Revolutionizing Quantum Communication: Direct Connections Between Multiple Processors
-
Agriculture and Food4 months ago
“A Sustainable Solution: Researchers Create Hybrid Cheese with 25% Pea Protein”
-
Diseases and Conditions4 months ago
Reducing Falls Among Elderly Women with Polypharmacy through Exercise Intervention
-
Albert Einstein4 months ago
Harnessing Water Waves: A Breakthrough in Controlling Floating Objects
-
Earth & Climate3 months ago
Household Electricity Three Times More Expensive Than Upcoming ‘Eco-Friendly’ Aviation E-Fuels, Study Reveals
-
Chemistry3 months ago
“Unveiling Hidden Patterns: A New Twist on Interference Phenomena”