While we try to keep things accurate, this content is part of an ongoing experiment and may not always be reliable.
Please double-check important details — we’re not responsible for how the information is used.
Artificial Intelligence
“Hopping into Action: MIT Researchers Develop Tiny Robot That Can Leap Over Obstacles with Ease”
A hopping, insect-sized robot can jump over gaps or obstacles, traverse rough, slippery, or slanted surfaces, and perform aerial acrobatic maneuvers, while using a fraction of the energy required for flying microbots.
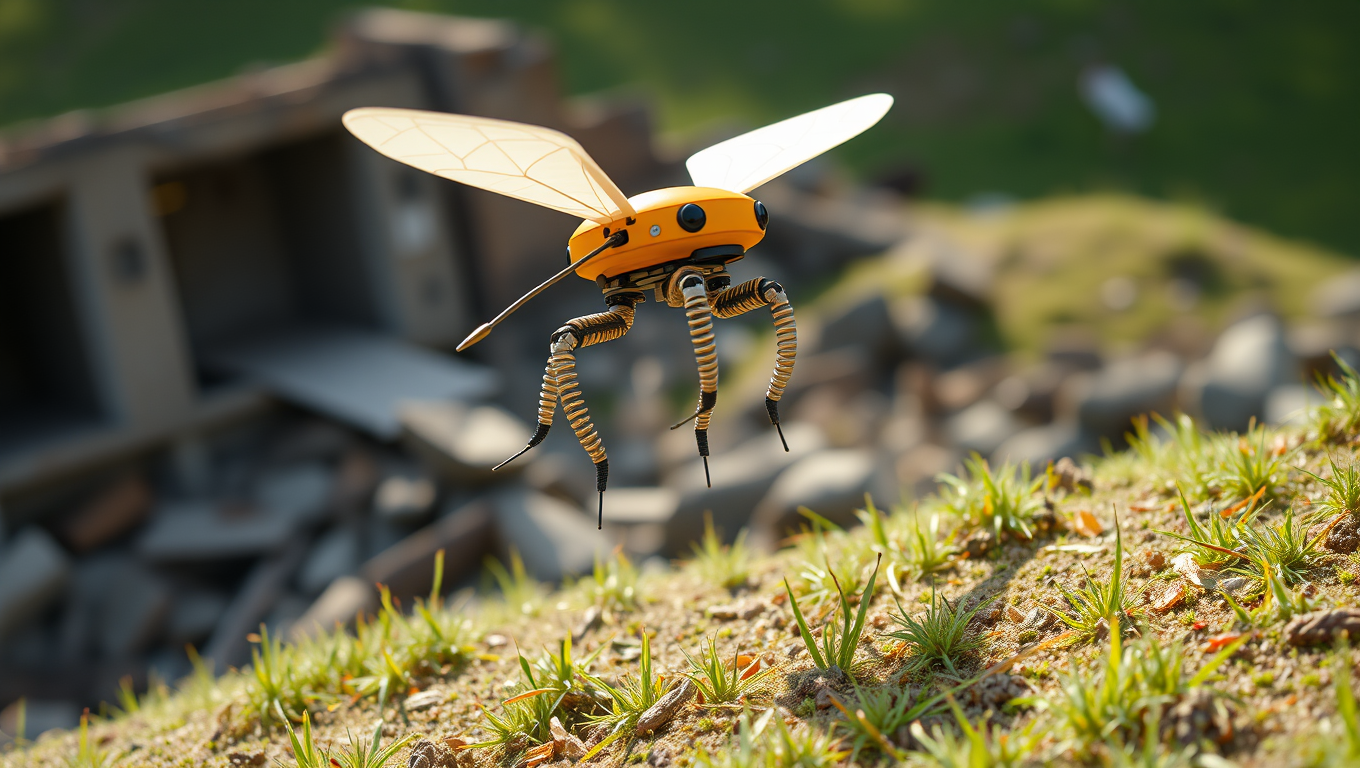
Artificial Intelligence
AI Uncovers Hidden Heart Risks in CT Scans: A Game-Changer for Cardiovascular Care
What if your old chest scans—taken years ago for something unrelated—held a secret warning about your heart? A new AI tool called AI-CAC, developed by Mass General Brigham and the VA, can now comb through routine CT scans to detect hidden signs of heart disease before symptoms strike.
Artificial Intelligence
Uncovering Human Superpowers: How Our Brains Master Affordances that Elude AI
Scientists at the University of Amsterdam discovered that our brains automatically understand how we can move through different environments—whether it’s swimming in a lake or walking a path—without conscious thought. These “action possibilities,” or affordances, light up specific brain regions independently of what’s visually present. In contrast, AI models like ChatGPT still struggle with these intuitive judgments, missing the physical context that humans naturally grasp.
Artificial Intelligence
“Future-Proofing Workers: How Countries Are Preparing for an AI-Dominated Job Market”
AI is revolutionizing the job landscape, prompting nations worldwide to prepare their workforces for dramatic changes. A University of Georgia study evaluated 50 countries’ national AI strategies and found significant differences in how governments prioritize education and workforce training. While many jobs could disappear in the coming decades, new careers requiring advanced AI skills are emerging. Countries like Germany and Spain are leading with early education and cultural support for AI, but few emphasize developing essential human soft skills like creativity and communication—qualities AI can’t replace.
-
Detectors3 months ago
A New Horizon for Vision: How Gold Nanoparticles May Restore People’s Sight
-
Earth & Climate4 months ago
Retiring Abroad Can Be Lonely Business
-
Cancer3 months ago
Revolutionizing Quantum Communication: Direct Connections Between Multiple Processors
-
Agriculture and Food4 months ago
“A Sustainable Solution: Researchers Create Hybrid Cheese with 25% Pea Protein”
-
Diseases and Conditions4 months ago
Reducing Falls Among Elderly Women with Polypharmacy through Exercise Intervention
-
Chemistry3 months ago
“Unveiling Hidden Patterns: A New Twist on Interference Phenomena”
-
Albert Einstein4 months ago
Harnessing Water Waves: A Breakthrough in Controlling Floating Objects
-
Earth & Climate3 months ago
Household Electricity Three Times More Expensive Than Upcoming ‘Eco-Friendly’ Aviation E-Fuels, Study Reveals