While we try to keep things accurate, this content is part of an ongoing experiment and may not always be reliable.
Please double-check important details — we’re not responsible for how the information is used.
Behavioral Science
Unlocking Cellular Secrets: How Deep Learning Revolutionizes Cytoskeleton Research
A research team has developed a groundbreaking deep learning-based method for analyzing the cytoskeleton — the structural framework inside cells — more accurately and efficiently than ever before. This advancement could transform how scientists study cell functions in plants and other organisms.
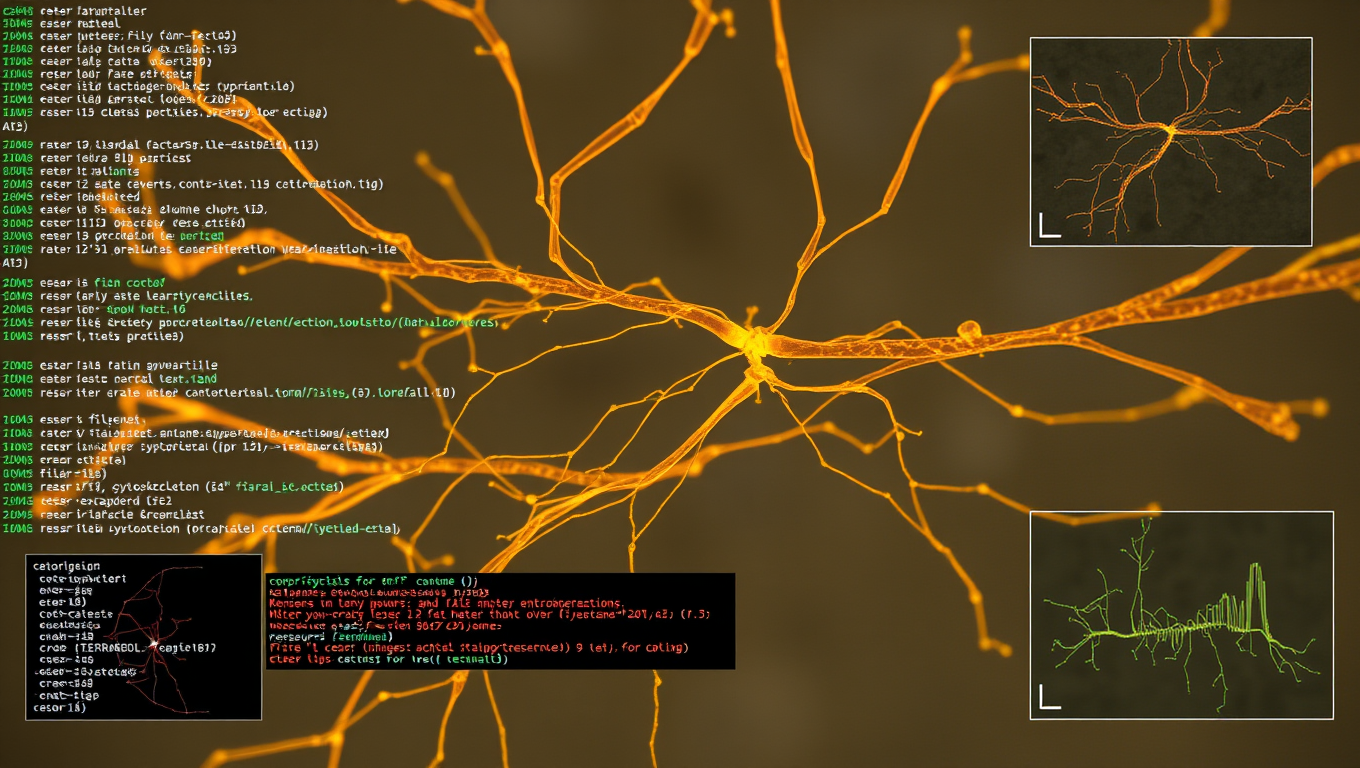
Behavioral Science
Satellite tracking of 12,000 marine animals reveals ocean giants are in trouble
A massive global collaboration has tracked over 12,000 marine animals from whales to turtles to create one of the most detailed movement maps of ocean giants ever assembled. The project, MegaMove, highlights how animal migrations intersect with fishing, shipping, and pollution, revealing alarming gaps in current ocean protections. Even if 30% of the oceans were protected, most critical habitats would still be exposed to threats.
Animals
Baboons’ Social Bonds Drive Their Travel Patterns, Not Survival Strategies
Researchers have discovered that baboons walk in lines, not for safety or strategy, but simply to stay close to their friends.
Behavioral Science
Unlocking the Secret to Staying Cool: A Breakthrough in Thermosensory Regulation
Researchers have identified a monoacylglycerol acyltransferase-coding gene named bishu-1. It is involved in the thermal responsiveness of cool temperature-sensing neurons by regulating ionotropic receptor expression, thereby maintaining the cool temperature avoidance behaviors in Drosophila larvae.
-
Detectors3 months ago
A New Horizon for Vision: How Gold Nanoparticles May Restore People’s Sight
-
Earth & Climate4 months ago
Retiring Abroad Can Be Lonely Business
-
Cancer3 months ago
Revolutionizing Quantum Communication: Direct Connections Between Multiple Processors
-
Agriculture and Food3 months ago
“A Sustainable Solution: Researchers Create Hybrid Cheese with 25% Pea Protein”
-
Diseases and Conditions4 months ago
Reducing Falls Among Elderly Women with Polypharmacy through Exercise Intervention
-
Chemistry3 months ago
“Unveiling Hidden Patterns: A New Twist on Interference Phenomena”
-
Earth & Climate3 months ago
Household Electricity Three Times More Expensive Than Upcoming ‘Eco-Friendly’ Aviation E-Fuels, Study Reveals
-
Albert Einstein4 months ago
Harnessing Water Waves: A Breakthrough in Controlling Floating Objects