While we try to keep things accurate, this content is part of an ongoing experiment and may not always be reliable.
Please double-check important details — we’re not responsible for how the information is used.
Computer Modeling
Unveiling New Disturbances in Hypersonic Flows
At hypersonic speeds, complexities occur when the gases interact with the surface of the vehicle such as boundary layers and shock waves. Researchers were able to observe new disturbances in simulations conducted for the first time in 3D.
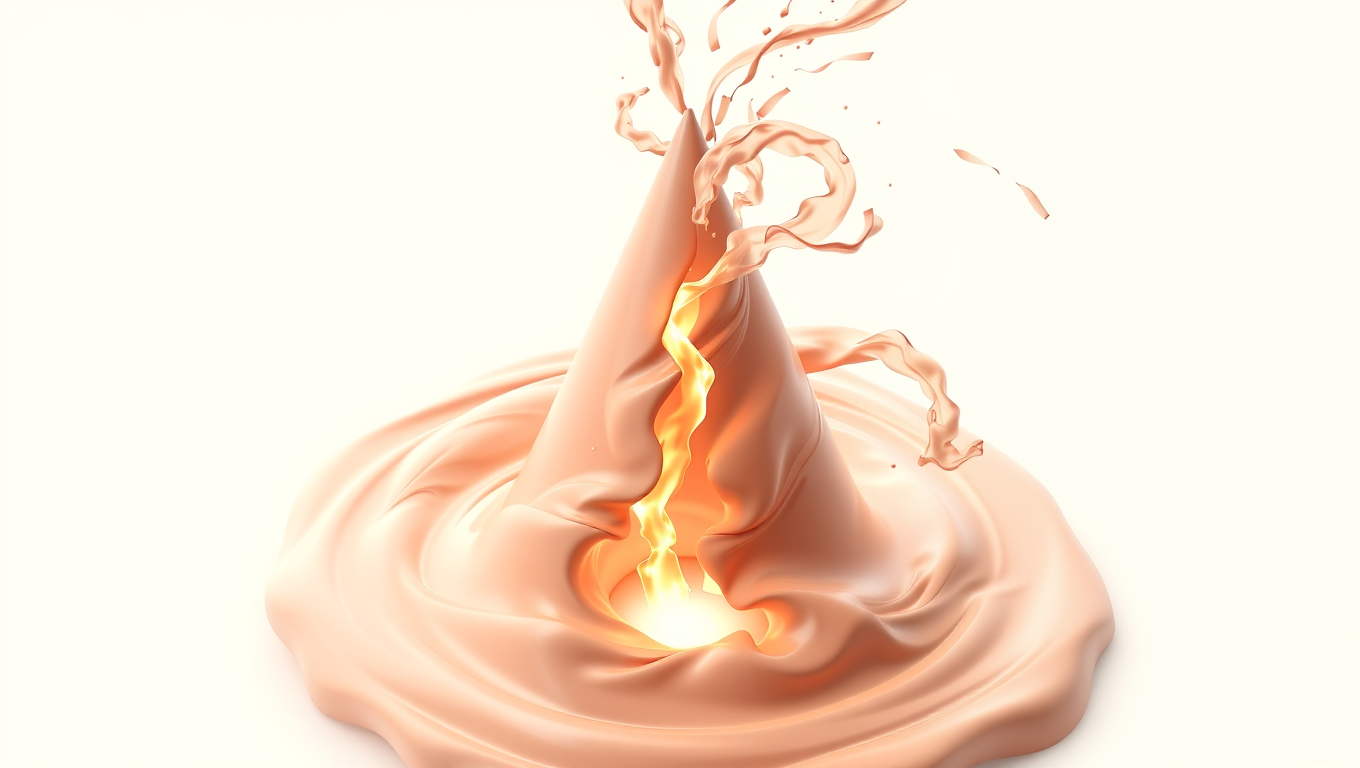
Computational Biology
A Quantum Leap Forward – New Amplifier Boosts Efficiency of Quantum Computers 10x
Chalmers engineers built a pulse-driven qubit amplifier that’s ten times more efficient, stays cool, and safeguards quantum states—key for bigger, better quantum machines.
Artificial Intelligence
AI Uncovers Hidden Heart Risks in CT Scans: A Game-Changer for Cardiovascular Care
What if your old chest scans—taken years ago for something unrelated—held a secret warning about your heart? A new AI tool called AI-CAC, developed by Mass General Brigham and the VA, can now comb through routine CT scans to detect hidden signs of heart disease before symptoms strike.
Computer Modeling
Harnessing True Randomness from Entangled Photons: The Colorado University Randomness Beacon (CURBy)
Scientists at NIST and the University of Colorado Boulder have created CURBy, a cutting-edge quantum randomness beacon that draws on the intrinsic unpredictability of quantum entanglement to produce true random numbers. Unlike traditional methods, CURBy is traceable, transparent, and verifiable thanks to quantum physics and blockchain-like protocols. This breakthrough has real-world applications ranging from cybersecurity to public lotteries—and it’s open source, inviting the world to use and build upon it.
-
Detectors2 months ago
A New Horizon for Vision: How Gold Nanoparticles May Restore People’s Sight
-
Earth & Climate4 months ago
Retiring Abroad Can Be Lonely Business
-
Cancer3 months ago
Revolutionizing Quantum Communication: Direct Connections Between Multiple Processors
-
Agriculture and Food3 months ago
“A Sustainable Solution: Researchers Create Hybrid Cheese with 25% Pea Protein”
-
Diseases and Conditions4 months ago
Reducing Falls Among Elderly Women with Polypharmacy through Exercise Intervention
-
Chemistry3 months ago
“Unveiling Hidden Patterns: A New Twist on Interference Phenomena”
-
Albert Einstein4 months ago
Harnessing Water Waves: A Breakthrough in Controlling Floating Objects
-
Earth & Climate3 months ago
Household Electricity Three Times More Expensive Than Upcoming ‘Eco-Friendly’ Aviation E-Fuels, Study Reveals