While we try to keep things accurate, this content is part of an ongoing experiment and may not always be reliable.
Please double-check important details — we’re not responsible for how the information is used.
Computer Modeling
Unveiling New Disturbances in Hypersonic Flows
At hypersonic speeds, complexities occur when the gases interact with the surface of the vehicle such as boundary layers and shock waves. Researchers were able to observe new disturbances in simulations conducted for the first time in 3D.
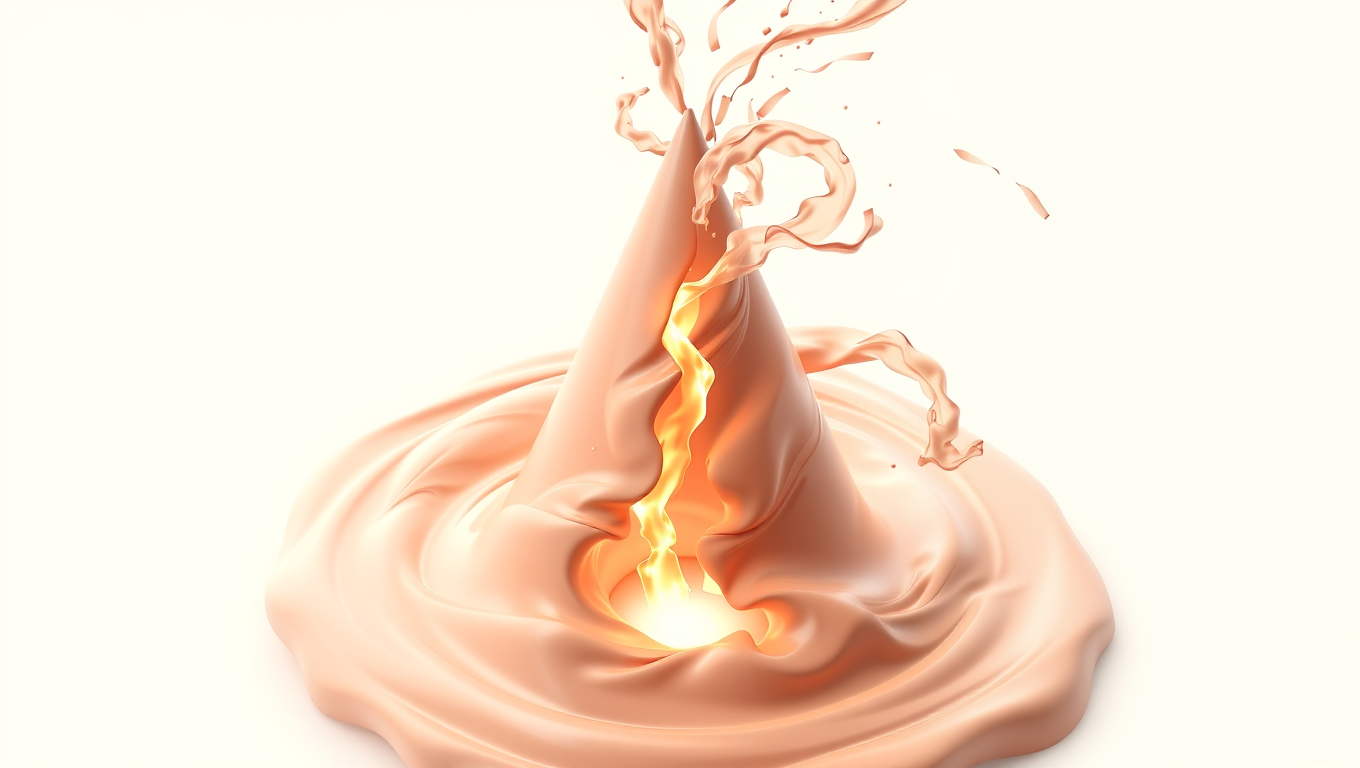
Artificial Intelligence
Scientists Uncover the Secret to AI’s Language Understanding: A Phase Transition in Neural Networks
Neural networks first treat sentences like puzzles solved by word order, but once they read enough, a tipping point sends them diving into word meaning instead—an abrupt “phase transition” reminiscent of water flashing into steam. By revealing this hidden switch, researchers open a window into how transformer models such as ChatGPT grow smarter and hint at new ways to make them leaner, safer, and more predictable.
Artificial Intelligence
The Quantum Drumhead Revolution: A Breakthrough in Signal Transmission with Near-Perfect Efficiency
Researchers have developed an ultra-thin drumhead-like membrane that lets sound signals, or phonons, travel through it with astonishingly low loss, better than even electronic circuits. These near-lossless vibrations open the door to new ways of transferring information in systems like quantum computers or ultra-sensitive biological sensors.
Computer Modeling
Scientists Crack Code to Simulate Quantum Computations, Paving Way for Robust Quantum Computers
A multinational team has cracked a long-standing barrier to reliable quantum computing by inventing an algorithm that lets ordinary computers faithfully mimic a fault-tolerant quantum circuit built on the notoriously tricky GKP bosonic code, promising a crucial test-bed for future quantum hardware.
-
Detectors3 months ago
A New Horizon for Vision: How Gold Nanoparticles May Restore People’s Sight
-
Earth & Climate4 months ago
Retiring Abroad Can Be Lonely Business
-
Cancer4 months ago
Revolutionizing Quantum Communication: Direct Connections Between Multiple Processors
-
Agriculture and Food4 months ago
“A Sustainable Solution: Researchers Create Hybrid Cheese with 25% Pea Protein”
-
Diseases and Conditions4 months ago
Reducing Falls Among Elderly Women with Polypharmacy through Exercise Intervention
-
Chemistry4 months ago
“Unveiling Hidden Patterns: A New Twist on Interference Phenomena”
-
Albert Einstein4 months ago
Harnessing Water Waves: A Breakthrough in Controlling Floating Objects
-
Earth & Climate4 months ago
Household Electricity Three Times More Expensive Than Upcoming ‘Eco-Friendly’ Aviation E-Fuels, Study Reveals